Avi Srivastava, Ph.D.
-
Assistant Professor, Genome Regulation and Cell Signaling Program, Ellen and Ronald Caplan Cancer Center
Srivastava is a computational biologist interested in advancing our understanding of the interplay among cellular and molecular modalities that determines cell fate.
Srivastava completed his undergraduate studies in computer science at the College of Engineering in Roorkee, India. He went on to earn his doctoral degree in Computational Biology from Stony Brook University, New York, and he then completed a postdoctoral fellowship at the New York Genome Center and New York University.
The Srivastava Laboratory
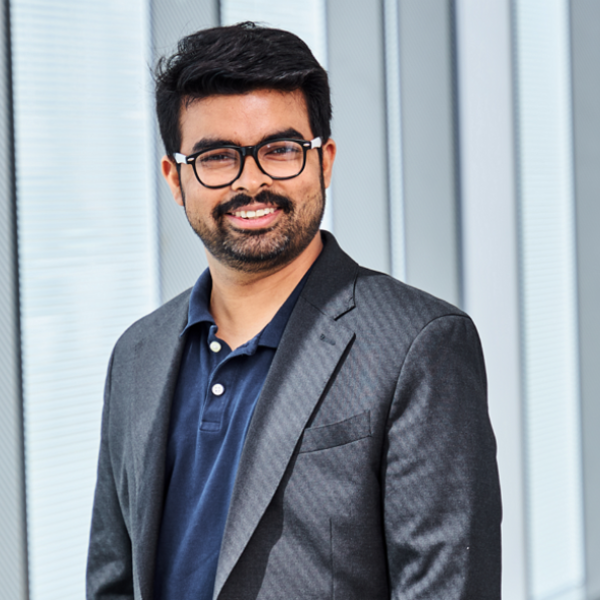
The Srivastava Laboratory
The Srivastava lab is dedicated to the holistic understanding of how the epigenome affects the transcriptional processes that determine cell fate. During hematopoiesis, multipotent hematopoietic progenitor cells navigate a series of regulatory steps to transform into various cell lineages essential for optimal function. Given the pivotal role that disrupted epigenomic regulatory patterns play in the progression of leukemia, exploring the chromatin dynamics within both unsuccessful (malignant) hematopoietic differentiation and healthy, successful differentiation allows us to decipher their underlying molecular mechanisms.
Our lab focuses on the intricacies of blood cell development, with a special emphasis on dysregulation in leukemia. We pursue this approach with a combined methodology that includes epigenetic, computational, and cancer biology analysis. By using state-of-the-science multimodal single-cell technologies and sophisticated, uncertainty-aware computational models, the Srivastava lab dissects chromatin state dynamics and their aberrations during cell differentiation.
-
Postdoctoral Fellow
Rajeev Ramisetti, Ph.D.
-
Research Assistant
-
We constantly seek out individuals with expertise in multi-disciplinary fields such as mathematics, biology, computer science, and related disciplines. Positions at multiple levels, including staff scientists and postdocs for both computational and experimental research, are available. Interested candidates should send a brief statement of their research interests (1-2 pages), CV, and their reference to asrivastava@wistar.org
Research
1. FAST AND EFFICIENT METHODS FOR BULK RNA-SEQ QUANTIFICATION
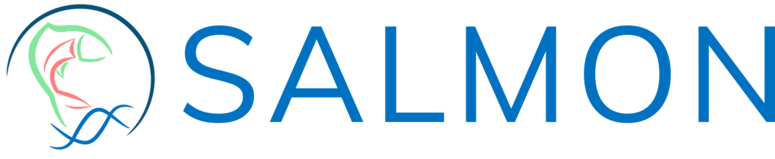
The accuracy of transcript quantification using RNA-seq data depends on many factors, such as the choice of alignment or mapping method and the quantification model. The alignment of sequencing reads to a transcriptome is a common and important step in many RNA-seq analysis tasks. While the choice of quantification model is important, considerably less attention has been given to the effect of various read alignment approaches on quantification accuracy. Thus, we investigated the influence of mapping and alignment of RNA-seq reads on the accuracy of transcript quantification and designed multiple novel alignment methodologies to overcome the shortcomings of lightweight approaches without incurring the computational cost of traditional end-to-end alignment.
* He, Dongze, et al. “Alevin-fry unlocks rapid, accurate and memory-frugal quantification of single-cell RNA-seq data.” Nature Methods 19.3 (2022): 316-322.
* Srivastava A., Malik L., Sarkar H., Zakeri M., Almodaresi F., Soneson C., Love MI., Kingsford C., & Patro R. (2020) “Alignment and mapping methodology influence transcript abundance estimation.” Genome Biology. 2020 Dec;21(1):1-29.
* Srivastava A., Sarkar H., Malik L. & Patro R. (2016) “Accurate, fast and lightweight clustering of de novo transcriptomes using fragment equivalence classes.” RECOMB-seq Conference. 2016 Apr 12.
* Srivastava A., Sarkar H., Gupta N. & Patro R. (2016) “RapMap: a rapid, sensitive, and accurate tool for mapping RNA-seq reads to transcriptomes.” Bioinformatics. 2016 Jun 15;32(12):i192-200.
2. UNCERTAINTY AWARE BAYESIAN METHODS IMPROVES SCRNA-SEQ QUANTIFICATION
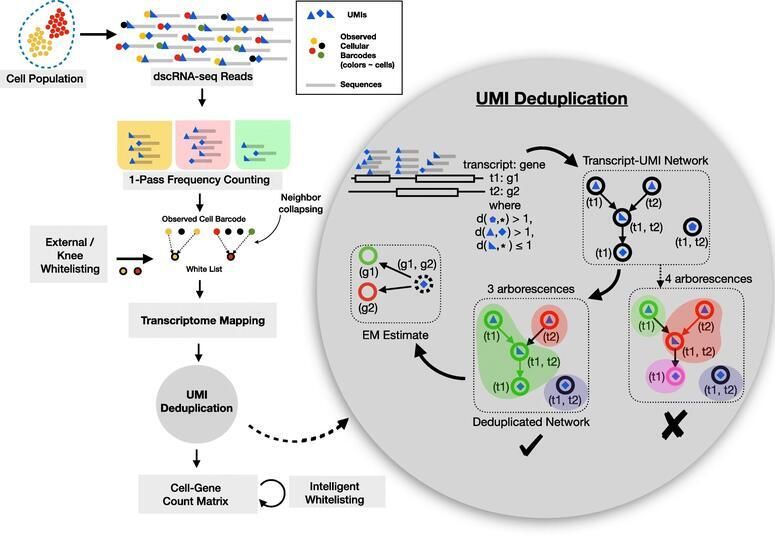
There has been a steady increase in the throughput of single-cell (sc)RNA-seq experiments, facilitating experimental assay of millions of cells. Droplet based scRNA-seq experiments have a large set of gene-ambiguous reads and can commonly account for a quarter of the sequenced data, which stays largely unused by quantification methods. We designed alevin, a fast end-to-end pipeline to process scRNA-seq data, performing cell barcode detection, read mapping, UMI deduplication, gene count estimation, and cell barcode whitelisting. Alevin’s approach to UMI deduplication provides an uncertainty-aware Bayesian model to account for reads that multimap between genes. This addresses the inherent bias in existing tools which discard gene-ambiguous reads and improves the accuracy of gene abundance estimates.
* Mu, Wancen, et al. “Airpart: interpretable statistical models for analyzing allelic imbalance in single-cell datasets.” Bioinformatics 38.10 (2022): 2773-2780.
* Soneson C., Srivastava A., Patro R. & Stadler MB. (2021) “Preprocessing choices affect RNA velocity results for droplet scRNA-seq data.” PLOS Computational Biology. 2021 Jan 11;17(1):e1008585.
* Srivastava A., Malik L., Smith T., Sudbery I. & Patro R. (2019) “Alevin efficiently estimates accurate gene abundances from dscRNA-seq data.” Genome biology. 2019 Dec;20(1):1-6.
* Zhu A., Srivastava A., Ibrahim JG., Patro R. & Love MI. (2019) “Nonparametric expression analysis using inferential replicate counts.” Nucleic Acids Research. 2019 Oct 10;47(18):e105.
3. COMPUTATIONAL METHODS FOR SINGLE-CELL ANALYSES
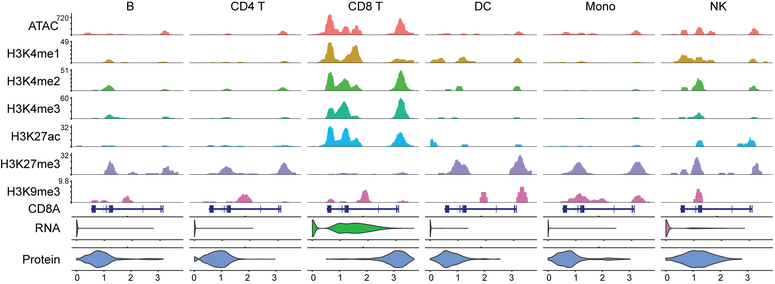
scRNA-seq data is being generated at an unprecedented pace, and the accurate estimation of gene-level abundances for each cell is a crucial first step in most scRNA-seq analyses. When pre-processing the raw scRNA-seq data to generate a count matrix, care must be taken to account for the potentially large number of multi-mapping locations per read. The sparsity of scRNA-seq data, and the strong 3’ sampling bias, make it even more challenging to disambiguate cases where there is no uniquely mapped read to any of the candidate target genes. We introduced a Bayesian framework for information sharing across cells within a sample or across multiple modalities of data to improve gene quantification estimates for scRNA-seq data.
* Hao, Yuhan, et al. “Dictionary learning for integrative, multimodal and scalable single-cell analysis.” Nature Biotechnology (2023): 1-12.
* Zhang, B.*, Srivastava A.*, Mimitou E., Stuart T., Raimondi I., Hao Y., Smibert P. & Satija R. (2021) “Characterizing cellular heterogeneity in chromatin state with scCUT\&Tag-pro.” Nature Biotechnology 40.8 (2022): 1220-1230.
* Stuart T., Srivastava A., Lareau C. & Satija R. (2021) “Single-cell chromatin state analysis with Signac.” Nature Methods (2021): 1-9.
* Srivastava A., Malik L., Sarkar H. & Patro R. (2020) “A Bayesian framework for inter-cellular information sharing improves dscRNA-seq quantification.” Bioinformatics. 2020 Jul 1;36(Supplement\_1):i292-9.
4. INTEGRATED ANALYSES OF THE EPIGENOME TO UNDERSTAND THE MOLECULAR BASIS OF HEMATOPOIETIC MALIGNANCIES
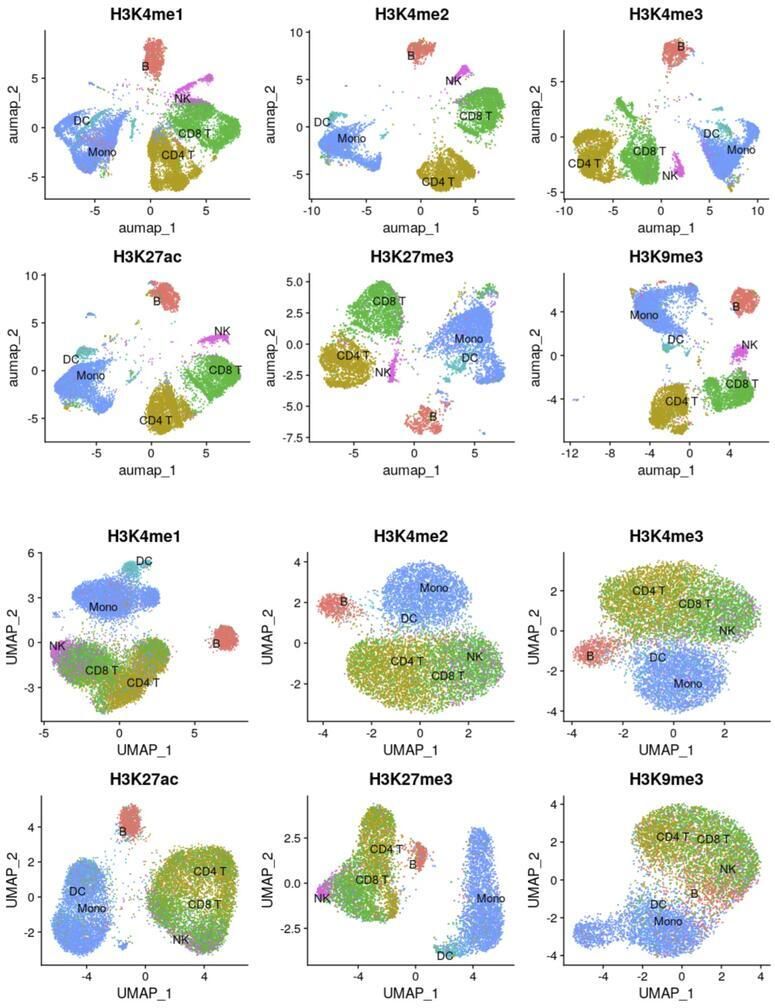
An impaired hematopoietic differentiation process underlies bone marrow malignancies like leukemia, but we still lack the mechanistic understanding of the sequence of regulatory events that misleads the differentiation process. Since epigenomic regulatory patterns are major features of leukemic development, understanding the chromatin dynamics of a failed (malignant) hematopoietic differentiation process can help define the molecular basis of leukemia. A prerequisite to such an understanding is a framework that allows investigation of the progressive changes in the activity of the regulatory elements (RE) during hematopoietic differentiation. Single-cell CUT&Tag (scCUT&Tag) technology is well-suited for such studies as RE activity through histone modification profiles can be investigated in a lineage-specific manner. Using scCUT&Tag we will investigate the RE and progressive changes in their activity during hematopoiesis. First, we will define a multimodal reference mapping framework for mouse hematopoiesis. This framework will allow us to integrate multiple histone modification profiles onto one reference and compare the chromatin states of the RE between a wild type (WT) and mouse model with loss of function in histone methyl transferase (HMT). Second, since HMTs regulate transcription through the interaction network of RE. We will define a chromatin state aware map that dynamically links REs across developmental trajectories. We will use this framework to investigate the changes in the interaction of REs due to HMT loss. Third, since the transcriptional state of a cell emerges from the underlying gene regulatory network (GRN), We will integrate single-cell gene expression data with histone modification profiles and extend it to define a chromatin state aware model of GRN. We will compare the WT and HMT loss experiments and define the differential GRN.
Srivastava Lab in the News
Selected Publications
Characterizing cellular heterogeneity in chromatin state with scCUT&Tag-pro
Zhang B, Srivastava A, Mimitou E, Stuart T, Raimondi I, Hao Y, Smibert P, Satija R. Characterizing cellular heterogeneity in chromatin state with scCUT&Tag-pro. Nat Biotechnol. 2022 Aug;40(8):1220-1230. doi: 10.1038/s41587-022-01250-0. Epub 2022 Mar 24. PMID: 35332340; PMCID: PMC9378363.
Alevin efficiently estimates accurate gene abundances from dscRNA-seq data
Srivastava A, Malik L, Smith T, Sudbery I, Patro R. Alevin efficiently estimates accurate gene abundances from dscRNA-seq data. Genome Biol. 2019 Mar 27;20(1):65. doi: 10.1186/s13059-019-1670-y. PMID: 30917859; PMCID: PMC6437997.
Alignment and mapping methodology influence transcript abundance estimation
Srivastava A, Malik L, Sarkar H, Zakeri M, Almodaresi F, Soneson C, Love MI, Kingsford C, Patro R. Alignment and mapping methodology influence transcript abundance estimation. Genome Biol. 2020 Sep 7;21(1):239. doi: 10.1186/s13059-020-02151-8. PMID: 32894187; PMCID: PMC7487471.
Dictionary learning for integrative, multimodal and scalable single-cell analysis
Hao Y, Stuart T, Kowalski MH, Choudhary S, Hoffman P, Hartman A, Srivastava A, Molla G, Madad S, Fernandez-Granda C, Satija R. Dictionary learning for integrative, multimodal and scalable single-cell analysis. Nat Biotechnol. 2023 May 25. doi: 10.1038/s41587-023-01767-y. Epub ahead of print. PMID: 37231261.
Alevin-fry unlocks rapid, accurate and memory-frugal quantification of single-cell RNA-seq data
He D, Zakeri M, Sarkar H, Soneson C, Srivastava A, Patro R. Alevin-fry unlocks rapid, accurate and memory-frugal quantification of single-cell RNA-seq data. Nat Methods. 2022 Mar;19(3):316-322. doi: 10.1038/s41592-022-01408-3. Epub 2022 Mar 11. PMID: 35277707; PMCID: PMC8933848.